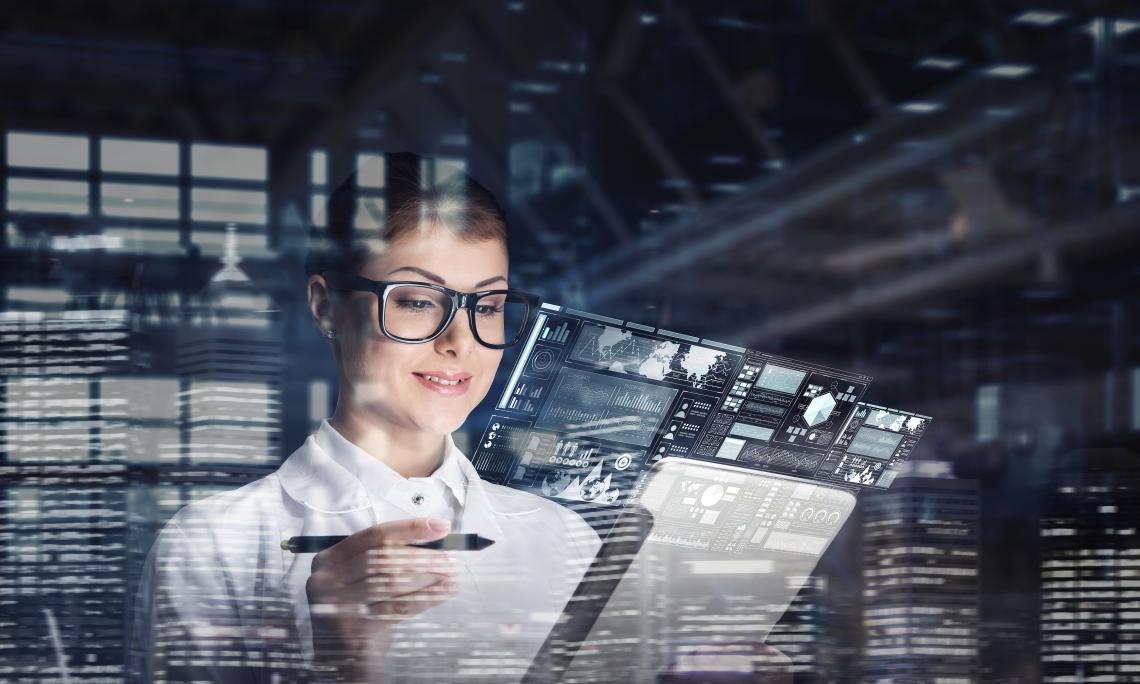
Machine learning (ML) refers to a method of data analysis that enables machines to make decisions essentially on their own. A visual tour of the process was presented by R2D3, detailing that it’s about identifying patterns in data through different methods. It is integral to the whole science of artificial intelligence (AI), as machines wouldn’t be able to demonstrate intelligence without learning from data.
The earliest known example of ML is playing checkers against a computer. There is no person secretly playing against you in real-time, as the programmer has enabled the computer to learn how to solve problems for itself. ML has come a long way from that and it's revolutionizing many technological aspects in a number of different industries. Here are some examples:
1. Healthcare - Increased precision
One of ML’s most beneficial uses is in healthcare, with Wired discussing that AI can fill the gaps left by a current global shortage in healthcare professionals. There are apps and wearable devices that can conduct patient diagnosis, monitor health and treatment, as well as enable people to adopt healthier lifestyles.
For example, notifying a person to take a certain number of steps in a day can bring about positive changes in overall wellness. AI is also being used by radiology departments as they can deliver faster and more accurate results. Robot doctors are also no longer unheard of, as they are now used to conduct keyhole surgeries.
2. Finance - Preempting issues
ML is making the finance industry more secure by detecting fraud early on before doing any major damage. CNBC reported on a scammer who duped 13 women he met on online dating sites and acquired $1.8 million until his arrest. Security that fends off fraudulent activities is a big advantage in a highly digital world.
Other aspects ML continues to advance are portfolio management, investment predictions, loans and underwriting. It is crucial in the trading sector, particularly in techniques like fundamental analysis, which is about predicting if the value of a stock or commodity will rise or dip. The same can be said for loans, as the system can learn how to collect/access data to expedite processes such as checking a person's credit history, background, etc.
3. Travel & Hospitality - More convenience
Ticketing and accommodation are the obvious sectors where ML is changing the travel & hospitality industry for the better. The tech automates the booking process while offering the most value for customers to increase loyalty. It also enhances security in airports with the use of facial recognition software.
ML can also find efficient routes for different modes of transportation as well as dictate proper pricing. Google is a perfect example, as a simple search about getting from one city to another will show you how long it might take. Different options and routes are also provided. You can search for flights, too, and estimates on fares will be provided in the search results.
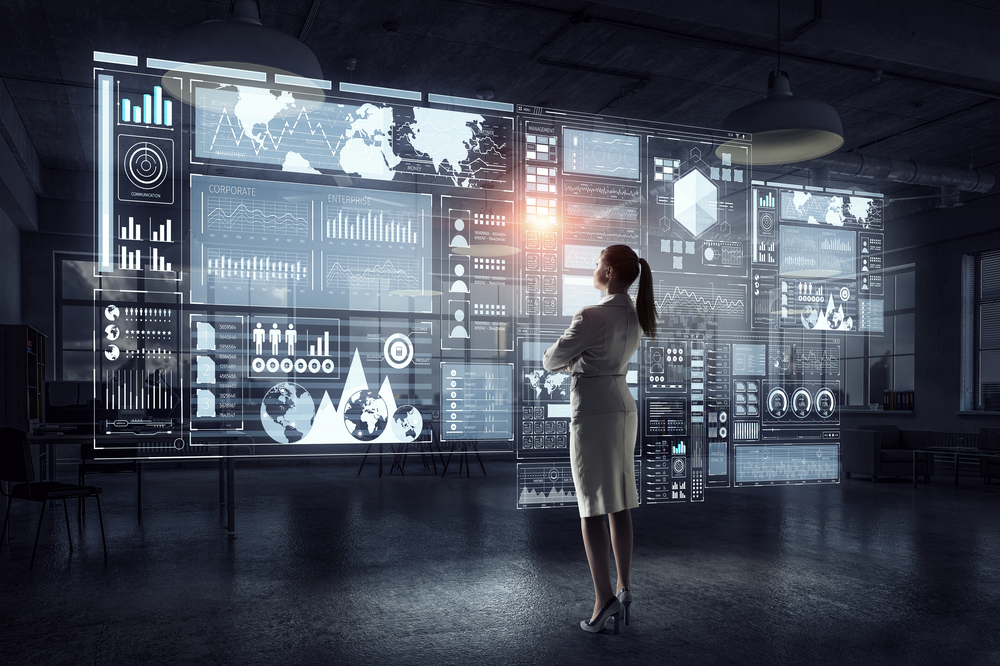
4. Retail - better personalization
AI and ML have become extremely important to the retail industry especially with a growing interest in online shopping. Brands are using the technology to track shopping habits, purchase histories, demand, and current market trends. It is also expected to change customer support with the use of smart chatbots. Here on Coding Girls we touched on the need for female experts in creating chatbots. One of the reasons is that they have better knowledge of the type of consumers that need customer support.
Chinese online retail giant Alibaba demonstrates these capabilities through their chatbot Dian Xiaomi. It helps with personalization by collecting consumer data through customer service. The chatbot serves an average of 3.5 million users every day, and it can answer queries from product information to return policies. Dian Xiaomi can even assess the emotions of a customer with the help of factors like tone and the words the consumer uses.
5. Supply Chain and Logistics - More efficient data collection
The tech has also opened up cost-saving opportunities for the supply chain and logistics department through improving data analysis. Yodel, one of Britain's largest parcel delivery companies, takes advantage of this improvement through its app that gives delivery information to customers. Yodel CIO Adam Gerrard explains how it works by saying, "if [customers] are number 85 on the current delivery tour, they know there’s a good chance that delivery is not going to happen for a couple of hours.”
This feature is now possible because machines learn how to analyze traffic data and road conditions. Verizon Connect UK details how advanced software contributes in selecting the best routes and making adjustments in real-time, which is the direction that Yodel is taking. In addition to cutting-edge programs, sensors and trackers are used to gather information and increase delivery efficiency. Drivers are welcoming the change too, as faster deliveries mean reduced fatigue and more time for non-business engagements. Louise Knight of London-based Lady & Van experiences this firsthand, as she occasionally works with charitable organizations like Women's Aid Federation of England.
6. Cyber Security - More robust software protection
Similar to fraud detection, cyber security also deals with increasing protection from network breaches. What cyber security companies do with ML and AI is use the data to detect hacking attempts and anomalies that can bring a whole system down. At the moment, it is not possible to protect all bits of data simultaneously in real-time, and this may not be achieved at all. What it means is that as the digital landscape evolves, there will always be new vulnerabilities that might be exploited by cyber criminals.
ML can assist further through three main processes, namely regression, classification and clustering. The first is about taking available data and comparing it with new data. It is followed by classification, which basically means marking sets of data (e.g. one may be dubious while the other is clean). The last is similar to classification, albeit it's more on grouping data sets with the same marks together. This three-step method can help cyber security experts assess threats and block them, as well as build protective systems against future attacks.
Have you been noticing these changes, too? What do you think of them? You may share your thoughts in the comments section!